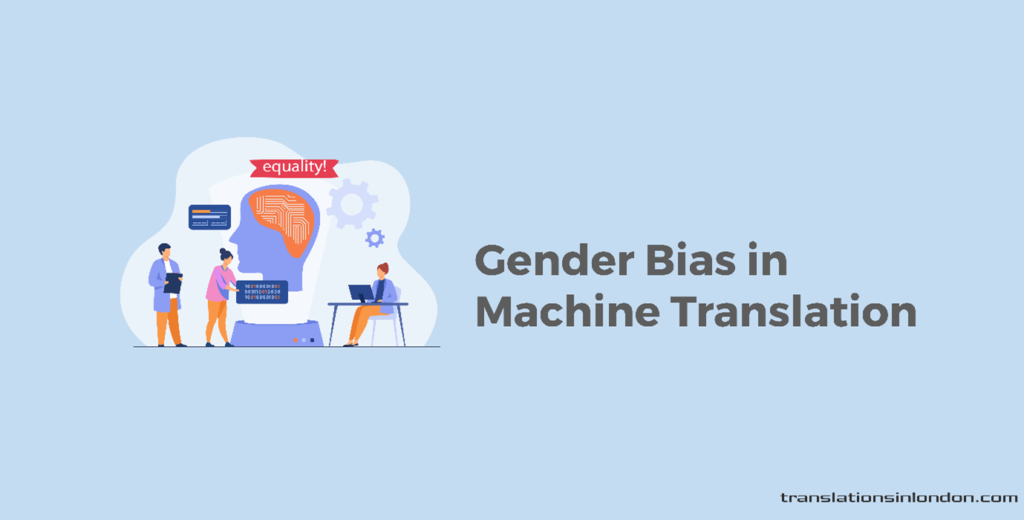
Although machine translation may have brought about many positives for language service providers and consumers alike, it is far from perfect.
There is an inherent problem with MT that we all need to have a conversation about; the issue of gender bias in Machine translation.
Take, for example, the previous issue of google translate assigning masculine pronouns to words like; doctor and feminine pronouns to nurse, or assigning certain activities to specific genders when attempting to translate gender-neutral languages.
Despite the growing awareness about gender inequality occurring worldwide, it is clear that more action needs to take place to fix gender bias in machine translation.
This post attempts to dissect gender bias in machine translation and the need to adopt diversity in the corporate space.
A multidimensional issue that requires robust solutions
The problem of gender bias in machine translation does not reside in just the incorrect translation of specific terms alone. It is much broader than that, and big tech companies seem to be part of it.
A good example is the practice of big tech companies like; Amazon, Apple, Microsoft on one hand, assigning female names (Alexa, Siri, and Cortana) to their voice assistant AI. While on the other hand, IBM’s supercomputer Watson bears a masculine, reinforcing existing gender stereotypes.
Although these companies claim that these personal assistants are genderless, most seem to have a female persona by default in many regions. And a good number do it for monetary reasons since scientific studies have established that people generally prefer the voice of a female to that of a male due to its warmth.
As such, there is a need for all active stakeholders to adopt a multilayered approach to solving issues of gender bias in machine translation.
Inherent societal bias could be the source of the problem
Making our way back to the issue of machine translation, we all must first identify the source of this bias. What are the root causes?
Some may argue that it is due to inherent human bias, which AI internalizes during the machine learning process. For example, the case of MT where bias is skewed against the female gender since the AI segment has high male domination.
Rather, Jonathan Davis, in his post, captures the problem succinctly. He says, “In many cases, this does not occur as the result of active bias by machine learning practitioners either when selecting their datasets or training their models. Rather, inherent societal biases, such as gender or ethnicity bias, manifest themselves in datasets which are essentially historical records of the given society. In turn, these datasets pass on their bias to the machine learning models that learn from them.”
But, it is common knowledge that getting rid of existing social bias is a lot easier said than done and it takes a long time.
Post-editing in MT plays a vital role in eradicating bias
From the surface, it is often easy to proffer a solution. Which, in this instance, some may say is eliminating these biases from the datasets.
But that does not solve the problem. These data are oftentimes a realistic representation of events. Once excluded, could result in an incomplete dataset which can cause large margins of error in a trained model.
One way forward is for language service providers, who, in a bid to take advantage of what MT offers while staying away from the scandals associated with gender bias, must adopt post-editing procedures into their process in order to reduce the impact of gender bias in their output to the barest minimum.
This is not the only solution. Stakeholders can reduce gender bias in MT by extensively training gender-sensitive datasets and employing the most efficient machine learning techniques to improve the accuracy of gender translation.
Perhaps, the most effective means of eliminating gender bias from machine translation is through the adoption of diversity in the entire ecosystem. From LSP teams to AI-based training samples and training personnel.
This solution is a more sustainable but long-termed approach that goes even as far as promoting other gender participation in AI technology to balance the already male-dominated space.
It should be worth noting that all participants must consider data concerning all prominent and emerging gender groups to forestall the re-emergence of this social issue.